🚀 How we're making AI work at Meilisearch
Struggling to make AI truly valuable at your company? Discover how we turned scattered AI usage into systematic success at Meilisearch, with a practical framework you can implement today.
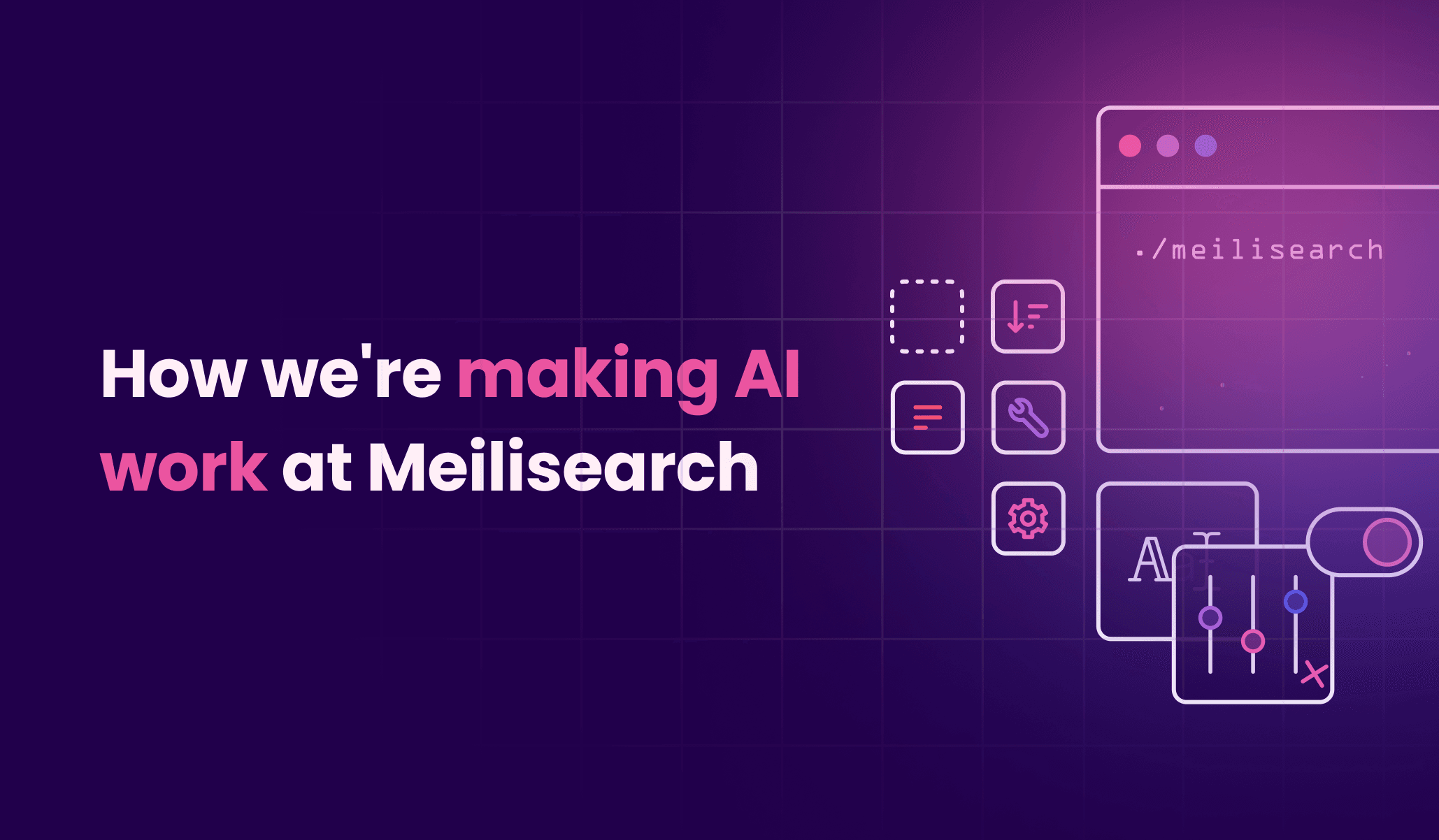
When we discovered that 70% of our team was already using AI tools daily—but with wildly varying results—we knew we needed a systematic approach. Not another vague "AI transformation" initiative, but a practical framework for separating AI hype from real value.
Here's the concrete system we developed to evaluate and implement AI tools across our company and what we've learned so far.
The surprising reality we uncovered
When we began our AI journey at Meilisearch, we expected to find pockets of adoption. Instead, we discovered something more interesting: universal experimentation but inconsistent value.
The adoption paradox
Our company-wide assessment revealed that every single team member had already experimented with AI tools, with an impressive 70% incorporating them into daily workflows.
But the numbers told only half the story:
Despite high adoption rates, team sentiment about AI's value showed surprising variation. Many were using the tools but questioning whether they were truly beneficial or just creating a new kind of busywork.
This gap between usage and perceived value became our first critical insight.
Four critical insights that changed our approach
Previously Shared Insights:
- 🧠 𝐃𝐨𝐞𝐬 𝐀𝐈 𝐛𝐞𝐜𝐨𝐦𝐞 𝐥𝐞𝐬𝐬 𝐯𝐚𝐥𝐮𝐚𝐛𝐥𝐞 𝐚𝐬 𝐲𝐨𝐮𝐫 𝐞𝐱𝐩𝐞𝐫𝐭𝐢𝐬𝐞 𝐠𝐫𝐨𝐰𝐬? How your relationship with AI changes at different levels of expertise.
- 🔍 Unlocking Our AI Knowledge-Sharing Blueprint: Understanding what people want to know to get started.
- 🤝 Preserving Human Connection in an AI-Enhanced Workplace: Will we lose what's special about our teams by bringing in AI?
Through in-depth interviews across all departments, we uncovered patterns that wouldn't have appeared in simple usage statistics:
- 🌿 Environmental impact: Our teams have raised thoughtful concerns about the environmental impact of AI.
- 🤔 The expertise paradox: As team members' domain expertise increases, their perception of AI's value often follows a U-curve. Beginners find AI helpful for learning, experts leverage it as a powerful accelerator, but those in the middle sometimes find it more hindrance than help.
- 🎯 The trust dilemma: AI's "inconsistent brilliance" creates a fundamental trust issue—sometimes transformative, sometimes bafflingly off-target—making teams reluctant to integrate it into mission-critical processes.
- ✨ Creative acceleration: Despite skepticism, nearly everyone reported dramatic improvements in creative processes—from eliminating writer's block to transforming editing workflows.
- 🔭 Prompt sophistication gap: Most team members were still using basic prompting techniques, unaware of how dramatically their results could improve with more advanced approaches.
This investigation revealed we weren't facing an adoption problem but a value extraction challenge. People were using AI tools but were not consistently getting meaningful results. We needed a framework to help teams identify where AI could actually create value—and where it was just adding complexity.
⚖️ Our solution: the AI value scoring matrix
Based on these insights, we developed a systematic framework to cut through the AI hype cycle. Rather than chasing the latest tools or models, we created a data-driven method to identify where AI could create genuine value for our specific context.
The value scoring matrix: Each potential AI use case is evaluated across multiple weighted dimensions aligned with our organizational priorities. This transforms the abstract question of "where should we use AI?" into a concrete, comparable set of scores.
Our Six Critical Dimensions:
Dimension | Weight | What We Assess | Why It Matters |
---|---|---|---|
Feasibility | 20% | Implementation difficulty with current skills/resources | Prevents us from chasing technically impressive but impractical applications |
Revenue Impact | 25% | Potential to increase top-line growth | Ensures AI initiatives contribute to business success |
Data Readiness | 15% | Availability and quality of necessary data | Many AI projects fail due to data issues, not technology limitations |
Time to Value | 15% | Speed to tangible results | Builds momentum with quick wins |
Risk | 10% | Potential challenges or downsides | Protects against unintended consequences |
Internal Impact | 15% | Number of people/teams benefiting | Prioritizes broad improvements over narrow optimizations |
Here's how we applied this framework to evaluate potential AI initiatives:
This systematic approach cut through subjective debates about which AI tools to adopt, replacing them with evidence-based decisions that aligned with our strategic priorities.
Early results: beyond the AI hype cycle
Our scoring matrix has already transformed how we approach AI at Meilisearch:
1. From endless options to strategic focus
Instead of drowning in the constant stream of new AI products, our teams now have a clear filter: "Does this tool address one of our high-scoring use cases?" This has dramatically reduced distractions and FOMO while increasing meaningful adoption.
2. Balanced investment: "Ferraris" and "cargo ships"
We're strategically balancing our implementation:
- "Ferrari" projects: Quick wins that generate excitement and momentum (e.g., automating our internal documentation updates)
- "Cargo ship" initiatives: Substantial efforts with transformative potential (e.g., reimagining our customer support workflow with AI assistance)
3. Creating a dedicated space for experimentation
One crucial lesson: AI adoption requires protected time. We've integrated dedicated "AI exploration blocks" into our work schedules and upcoming offsite, focusing specifically on our highest-scoring use cases.
4. Addressing real concerns
The scoring matrix naturally incorporates ethical considerations through the risk dimension. We've operationalized this by:
- Favoring smaller, more efficient models when possible
- Prioritizing open-source options that allow us to run models locally
- Building in time for human review of AI-generated content
The framework in action: a case study
After applying our scoring matrix to use cases identified through internal discussions, we quickly surfaced high-impact opportunities. One standout example was improving our code review process—specifically, reducing time to first review for pull requests (PRs).
This use case scored highly across multiple dimensions:
- High feasibility with existing tools
- Direct impact on development velocity
- Clear time-to-value metrics
- Broad internal impact across engineering teams
We implemented CodeRabbit, an AI-powered code review assistant that provides immediate PR feedback. While maintaining our essential human review process, the tool identifies common issues and provides automated PR descriptions. After a two-week trial period, we measured both quantitative metrics and gathered team feedback.
The results validated our scoring framework's prediction:
- Decreased average time to first review
- Improved PR documentation quality
- Positive team adoption and engagement
- Cumulative time savings across the engineering organization
What's next: your turn
We're sharing this framework because we believe it can help other organizations cut through AI hype and focus on value creation.
The key to successful AI adoption isn't about having the fanciest tools—it's about having the clearest framework for identifying where AI truly adds value in your specific context.